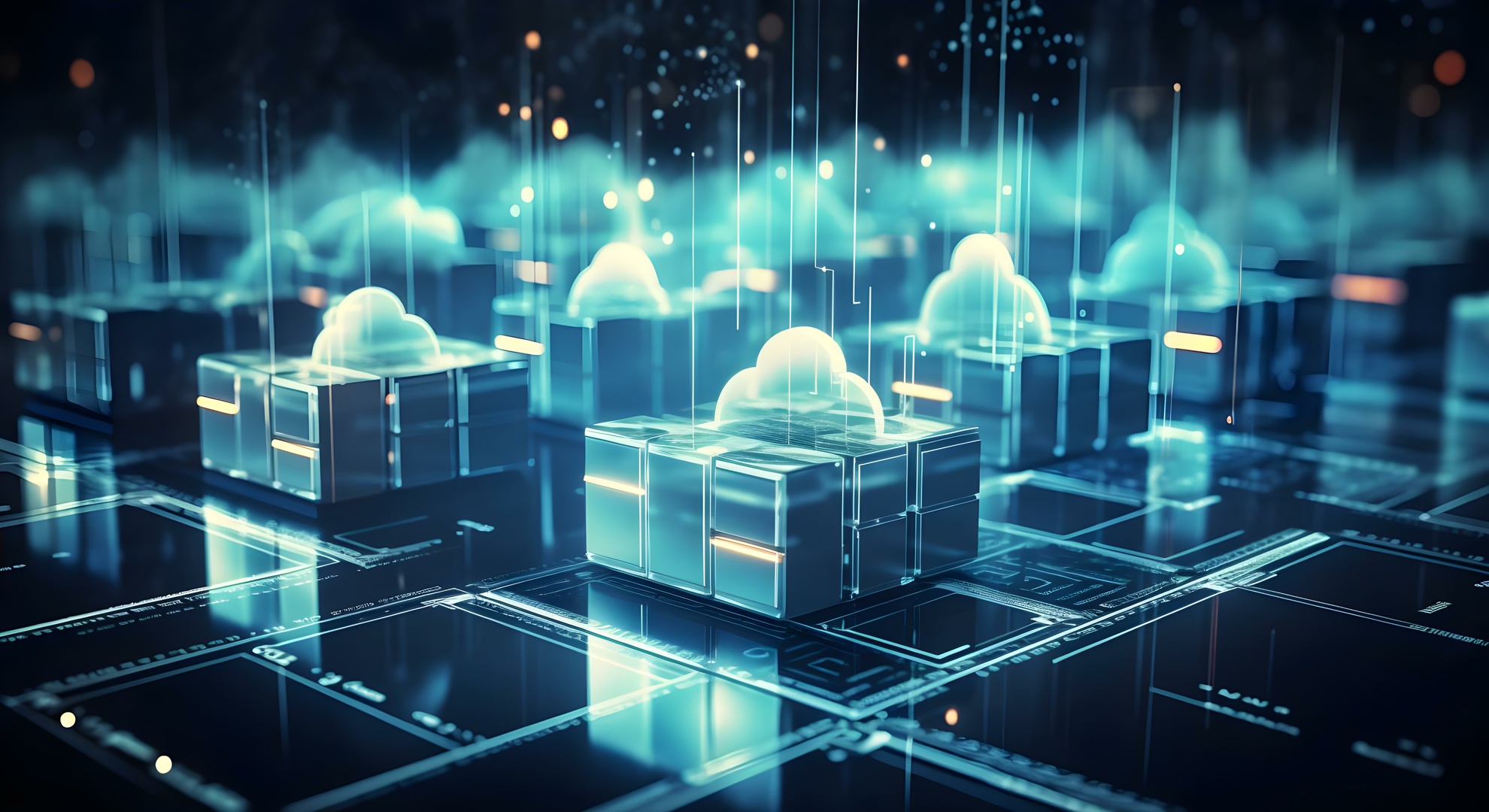
13 Dec What is edge computing? How it stores data and saves bandwidth
In the midst of the digital revolution, it can often be challenging to keep up with advancements and identify which are truly disruptive or poised to become so. Edge computing is one of these breakthroughs—a decentralised processing model that places data management and analysis closer to the points where the data is generated, rather than relying exclusively on servers or cloud infrastructures.
How can edge computing be defined?
Edge computing is a working model or conceptual approach that frames how processes function in the world of computing. According to this approach, all logical operations, data-related processes, and their storage and management are positioned as close as possible to the devices or servers nearest to the user or source of the information.
Edge computing becomes clearer when contrasted with traditional models, which typically send information to remote data centres far from the user. Processing data at the “edge”—its literal translation—reduces latency and increases the efficiency of technological infrastructures that adopt this model. This is particularly significant given the current exponential growth in data consumption and the volume demanded by technologies such as artificial intelligence, smart networks, or the Internet of Things (IoT), to name just a few examples.
How does edge computing store data and save bandwidth?
In edge computing, data is stored in distributed nodes, which can include local devices, regional servers, or network gateways. These nodes have the capacity to store and process data at the point where it is generated, eliminating the need to send everything to a centralised data centre. This approach offers several advantages, as mentioned earlier: it reduces latency, optimises bandwidth—since only relevant information is transmitted to servers or the cloud—and enhances security by allowing sensitive information to remain within local networks, thereby reducing risks.
Key use cases
As edge computing focuses on efficiency, resource optimisation, and faster data processing, its practical applications are virtually limitless. Some notable use cases include:
- Cybersecurity: Edge computing enables real-time threat detection by conducting local analysis on devices connected to a network. This simplifies the protection of systems and devices without relying on external connections.
- IoT and smart cities: Edge computing shifts the workload to local infrastructures, allowing data from local sensors to manage traffic systems, lighting, and waste collection efficiently. This autonomy reduces dependence on external agents for those administering edge systems.
- Healthcare: In hospitals, medical devices can analyse vital data instantly, improving patient care without waiting for responses from hierarchically superior structures or remote locations relative to the hospital’s premises.
- Retail: In physical stores, edge computing enables real-time personalised offers based on customer behaviour. This allows businesses to adapt to local trends and dynamics without being bound by corporate policies that may not align with the local audience’s preferences.
All the use cases mentioned, along with many others, can benefit even further when edge computing interacts with artificial intelligence. Running algorithms on local servers, making predictions based on behaviour analysis or failure patterns, conducting heuristic analyses, and personalising experiences make technology not only faster and more efficient but also more relevant and accessible for users—ultimately, the true beneficiaries of any technological advancement.